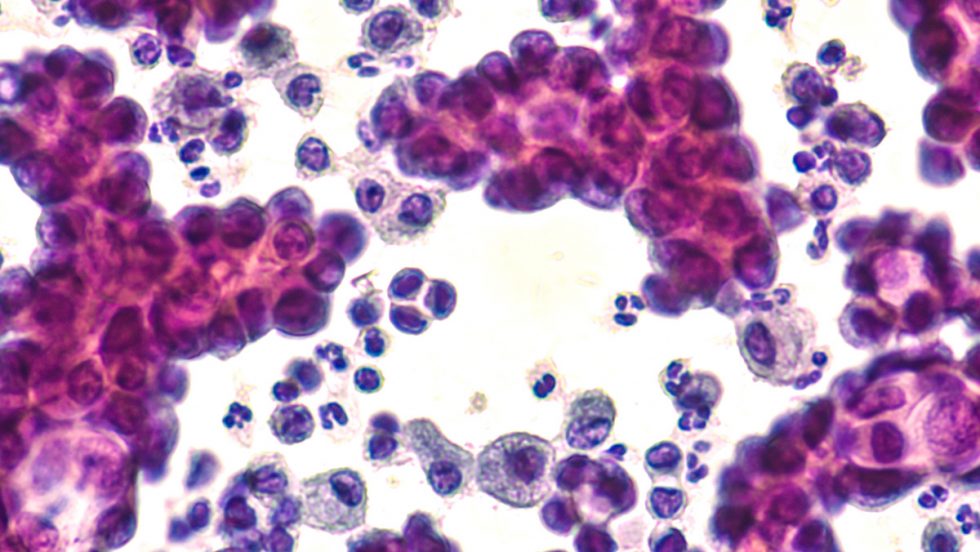
When patients are given the diagnosis no one ever wants to hear—that they have cancer—the first questions most will ask are about their prognosis.
“Will I beat this?” “How long do I have?”
These types of questions are also among the most challenging for physicians to answer with accuracy. Uncertainty related to prognosis can also impact decision-making when it comes to treatment.
Zahra Sedighi-Maman, PhD, assistant professor of decision sciences and marketing in the Adelphi University Robert B. Willumstad School of Business, has conducted research that aims to harness the power of technology—machine learning—to give healthcare professionals a new tool for predicting the survivability of lung cancer, the leading cause of cancer-related deaths according to the World Health Organization. She and a colleague from Georgetown University recently published “An Interpretable Two-Phase Modeling Approach for Lung Cancer Survivability Prediction,” in Machine Learning Methods for Biomedical Data Analysis, a special issue of the journal Sensors, which creates a two-phase data analytic framework leveraging both lung cancer survival status and survival-length predictions in an interpretable way.
“My research focus has been driven by my motivation to improve clinical care for patients by analyzing complex data in a critical area such as healthcare,” said Dr. Sedighi-Maman. “The data-driven findings from our research may help physicians in their decision-making process when it comes to lung cancer diagnosis and special treatments using interpretable analytical models.”
Dr. Sedighi-Maman and her co-author developed their data analysis framework using several methods to extract important variables, such as age, surgery status and the stage of cancer, that help predict lung cancer survival. In Phase I of the research, they used several prediction models in order to classify patients by the likelihood they will remain alive at the 0.5-, 1-, 1.5-, 2-, 2.5- and 3-year time points, and in Phase II they predict the number of survival months within three years using recent Surveillance, Epidemiology, and End Results Program data from the National Cancer Institute.
To gain the most insight from complex and varying information gained through data mining, such as grade of cancer, stage of cancer, or race, the authors used the “one-hot encoding method” that transformed it into binary variables. In doing so, they are able to properly interpret the impact of individual levels of each of these variables.
Dr. Sedighi-Maman explains, “By doing this, we can compare the effects of Grade I and Grade III cancer on patients’ survival length individually, rather than simply stating that grade of cancer is important.”
And because the predictions that can be made are only as good as the data going in, the authors took care to conduct extensive “data cleansing” to remove unknown/missing values, duplicate variables and correlated features in the lung cancer data set.
In creating their data analysis frameworks, Dr. Sedighi-Maman and her co-author proved that simpler, interpretable models like the General Linear Model perform just as well as more complex models. She says that in doing so, they were able to quantify the impact of individual important variables like age, surgery status and stage of cancer on lung cancer prediction in a way that physicians and clinical researchers can interpret and apply to their approaches to treating patients.
Takeaways on Lung Cancer Survivability
Some key findings to emerge from Dr. Sedighi-Maman’s research may help to inform the course of action medical practitioners take when developing plans of care for patients diagnosed with lung cancer. Among them:
- Regional cancer is significantly correlated with a patient’s odds of survival across all time points. Localized cancer is also a significant feature that positively affects a patient’s survival status, especially at early time points. In cases of both localized or regional spread, patient survival is expected to be extended by 4.71 or 2.59 months, respectively.
- Conversely, cancer that has metastasized to the liver decreases survivability. Metastasis to the liver is the top significant feature that negatively affects the number of survival months.
- If surgery is performed on a primary site, a patient’s odds of survival are higher on average across survival time points.
- Having the primary site of the lung cancer in the upper lobe lung is associated with higher odds of survival for several time points.
- For every additional year in age (age at diagnosis), a patient who is not anticipated to survive is expected to live 1.24 months less on average, holding other features constant.
Dr. Sedighi-Maman and her co-author caution that other potential factors, like a patient’s lifestyle—diet or smoking behaviors or prior medical/drug history—may impact lung cancer survivability, but they hope that their simple yet interpretable models might enable physicians to provide more informed healthcare by prioritizing the most important factors related to lung cancer survivability.
“We hope that our research will encourage clinical practitioners to implement data analytics in healthcare decision-making.”